Slicenet PoC proposal with ETSI ENI on Predictive Fault Management of end to end (E2E) Multi-Domain Network Slice (NS)
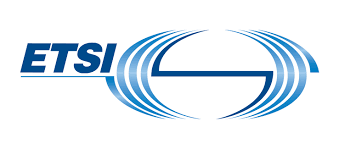
In this PoC, where an E2E NS is composed of several NS, the prediction of a faulty behaviour on one of these NS is performed by making use of AI/ML mechanisms and will trigger policy based actions that will proactively make the necessary modifications on that NS to guarantee the E2E NS availability with the committed SLA.
SliceNet Standards Contribution to ETSI Generic Autonomic Networking Architecture
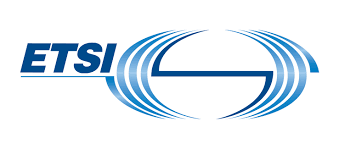
This technical report presents a plausible approach to implementing Federated GANA Knowledge Planes (KPs) Platforms for E2E Multi-Domain Federated Autonomic Management and Control (AMC) of Slices in NGMN E2E 5G Architecture, using components prototyped and implemented in the SliceNet Project.The work produces and leverages a mapping of architectural components for autonomic network management & control developed/implemented in the SliceNet Project to the ETSI TC INT AFI Generic Autonomic Networking Architecture (GANA) model – an architectural reference model for autonomic networking, cognitive networking and self-management. The mapping identifies the components that can be used to implement specific GANA Functional Blocks (FBs) for Autonomics and their associated Reference Points (Rfps), while providing the illustrations that help implementers of GANA autonomics in 5G networks.
Contribution 1 to ITU-T Focus Group ML5G under SG13
This is a new ITU-T Focus Group ML 5G draft recommendation, under ITU-T SG13. The document is registered under ML5G-I-247 (formerly ML5G-I-230) number with the title – “Machine learning based end-to-end network slice management and orchestration”. This Recommendation provides the framework and requirements of machine learning based end-to-end network slice management and orchestration in multi-domain environments.
This contribution is available to members of ML5G here: extranet.itu.int
Contribution 2 to ITU-T Focus Group ML5G under SG13
The document is registered in ITU-T Focus Group ML5G under ML5G-I-242-R1 (formerly ML5G-I-231) with the title “Vertical-assisted Network Slicing Based on a Cognitive Framework”.
This contribution proposes a new framework that enables vertical QoE-aware network slice management empowered by machine learning technologies.
This contribution proposes a new framework that enables vertical QoE-aware network slice management empowered by machine learning technologies.
This contribution is available to members of ML5G here: extranet.itu.int
Contribution 3 to ITU-T Focus Group ML5G under SG13
The document is registered in ITU-T Focus Group ML5G under ML5G-I-198-R1 (formerly ML5G-I-198) with the title “Anomaly prediction and integration for eHealth use case based on vertical feedback”.
This contribution presents a ML model that aims to forecast the signal degradation using vertical feedback. Proposed anomaly detection model serves as an intelligent QoE sensor by analysing the data samples related to perceived quality of the service called “quality of perception” (QoP) optimal QoE levels. By forecasting the perceived signal strength degradation, the network maintainers may
be alerted in advance and the issue could be solved before it occurs and this will allow maintaining the perceived quality of the slices’ services but also it can allow the vertical to supervise its slice and to interact with the network. This model is integrated in eHealth Use Case.
This contribution presents a ML model that aims to forecast the signal degradation using vertical feedback. Proposed anomaly detection model serves as an intelligent QoE sensor by analysing the data samples related to perceived quality of the service called “quality of perception” (QoP) optimal QoE levels. By forecasting the perceived signal strength degradation, the network maintainers may
be alerted in advance and the issue could be solved before it occurs and this will allow maintaining the perceived quality of the slices’ services but also it can allow the vertical to supervise its slice and to interact with the network. This model is integrated in eHealth Use Case.
This contribution is available to members of ML5G here: extranet.itu.int
Contribution 4 to ITU-T Focus Group ML5G under SG13
The document is registered in ITU-T Focus Group ML5G under ML5G-I-199 with the title “Noisy neighbour detection and integration in a virtualized infrastructure”.
This contribution presents a ML model that aims to detect noisy neighbours in a virtualized infrastructure. Proposed noisy neighbour analytical workflow to determine if a Virtual Network Function (VNF) instance belonging to a slice is being subject to CPU noise coming from another VNF instance collocated into the same physical server or if the VNF is being overloaded due to its internal processes. This model is integrated in Smart City Use Case that implements Intelligent Public Lighting system.
This contribution presents a ML model that aims to detect noisy neighbours in a virtualized infrastructure. Proposed noisy neighbour analytical workflow to determine if a Virtual Network Function (VNF) instance belonging to a slice is being subject to CPU noise coming from another VNF instance collocated into the same physical server or if the VNF is being overloaded due to its internal processes. This model is integrated in Smart City Use Case that implements Intelligent Public Lighting system.
This contribution is available to members of ML5G here: extranet.itu.int
SliceNet Standards Contribution on the IETF Definition of Transport Slice
SliceNet system architecture and definition of Slice has been discussed with Ericsson representatives at IETF to start and contribute to the Internet-Draft “IETF Definition of Transport Slice”.
Internet-Drafts are working documents of the Internet Engineering Task Force (IETF). The draft is still active being worked on by the IETF [Teas] Network Slicing Design Team.
Internet-Drafts are working documents of the Internet Engineering Task Force (IETF). The draft is still active being worked on by the IETF [Teas] Network Slicing Design Team.
The current live version can be found at V05-draft-nsdt-teas-transport-slice-definition.